Check out the other tools in our employee engagement suite designed to help you collect feedback, uncover insight, and take action with confidence and ease.
EMPLOYEE ENGAGEMENT SURVEY SOFTWARE
Understand the big picture to boost employee engagement.
Every employee's voice is important—but capturing these voices without a trusted strategy or efficient tools is counterproductive. Your employee engagement survey software should help you maximize employee participation, give you an accurate baseline, and actionable insights that improve employee experiences and performance.
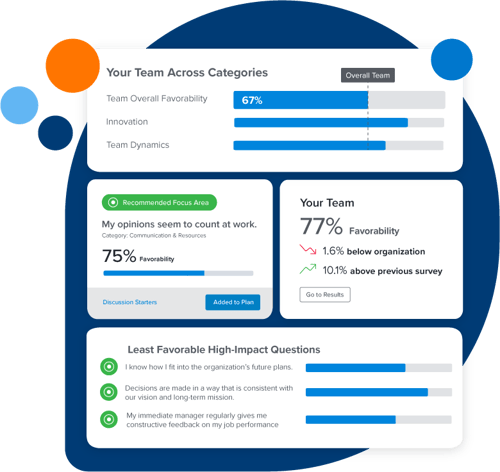
EMPLOYEE ENGAGEMENT SURVEY PLATFORM
Measure employee engagement easily and accurately.
Our trusted employee engagement survey software makes collecting survey responses, analyzing results, and acting on meaningful company-wide feedback effortless for everyone involved.
Survey design you can trust
When it comes to measuring employee engagement, any old survey won’t do. Our 30-item engagement survey is designed by talent experts and researchers immersed in best practices and the ever-changing workplace.
- Scientifically validated e9 model: proven 3-pronged model with nine tested questions that measure employee engagement levels
- Research-backed questions: specific questions help you understand how to drive engagement across your organization
- 6-point agreement scale: standard survey measurement improves the quality of your survey data
- Integrated, open-ended questions: survey comments to easily collect tangible examples of what to start, stop, or continue
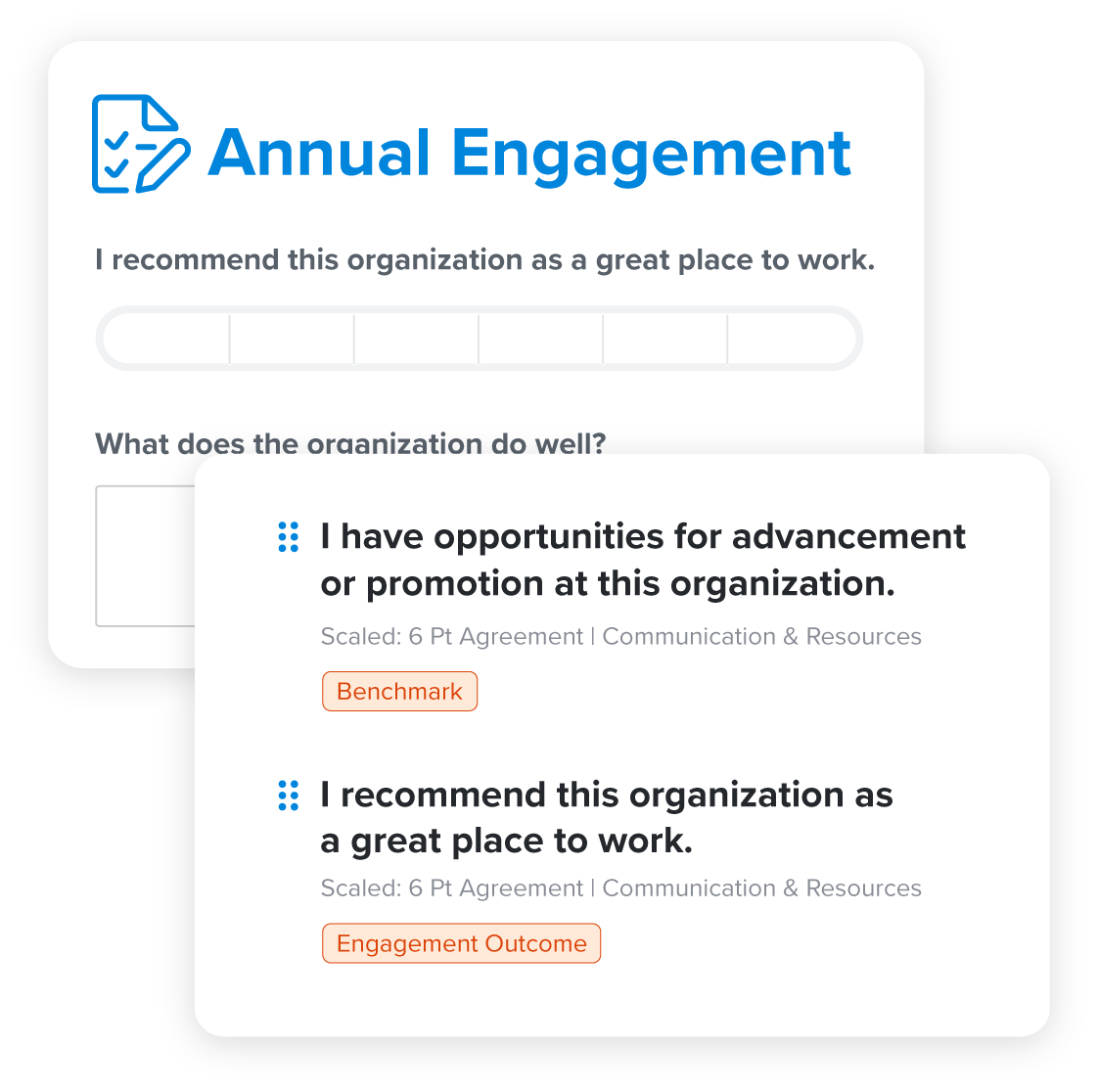
Identify trends and share results
Easily understand and share survey results with powerful analytics and reporting. Our custom demographic filters and interactive data visualizations allow you to analyze and view your organizational data any way you want.
Simplified dashboards and reporting features help highlight only the most valuable insights for managers and teams.
Add context with unparalleled benchmarks
Elevate your culture as a competitive advantage with our benchmarks. As the employee engagement survey software behind America’s Best Places to Work contest, our employee engagement data enables you to compare your results to thousands of organizations in your industry and size category.
- North America’s largest database: 10,000+ organizations and 1+ million surveys completed annually
- Best-in-class benchmarks: comparisons against companies who win Best Places to Work awards
- Relevant annual updates: new benchmarks to reflect current workplace trends help you stay up-to-date
- Available in-tool: benchmarks are accessible within analytics and can be incorporated into action plans
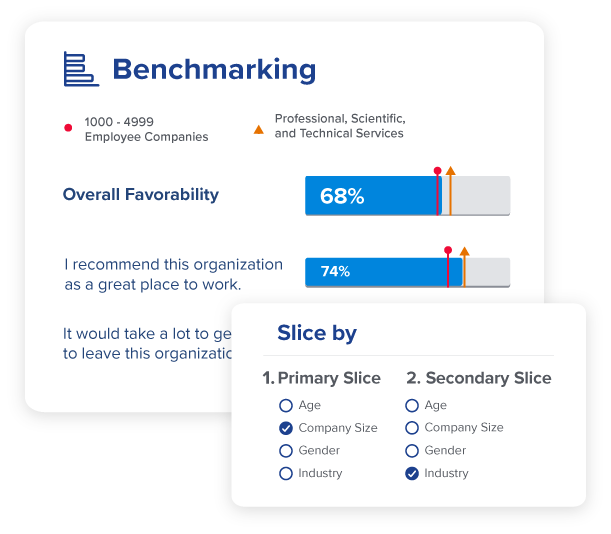
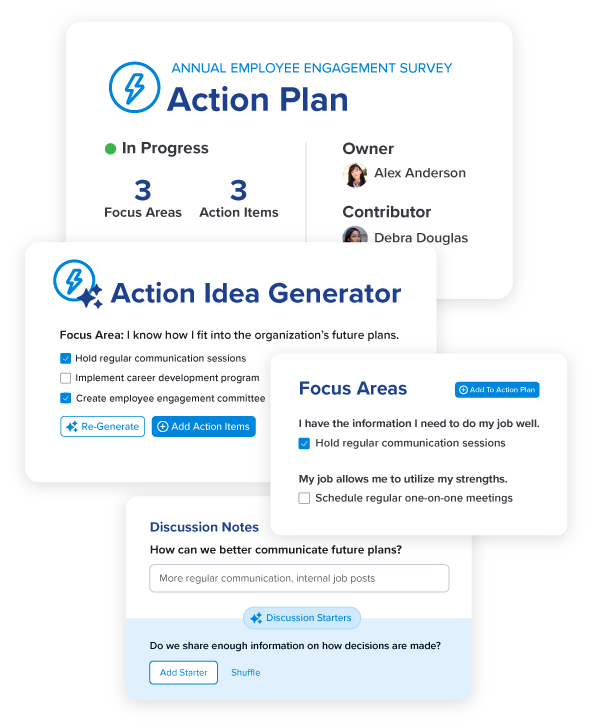
Enable your managers with easy access
Managers are on the front lines of engagement but are often under-equipped to play a leading role. Our employee engagement survey software makes it easy for managers to understand results, take targeted action, and stay accountable for improvement.
- Team report & walkthrough: guided overview of team-level engagement results
- Recommended focus areas: tagged categories and items that show managers where to focus
- ✨AI-powered discussion starters: Expert-informed, recommended prompts for meaningful engagement conversations within your team
- ✨AI-powered action ideas: Action recommendations guided by experts to reduce time-to-action and increase impact
Hold managers and teams accountable for action
Insight is useless if you don’t take action. Our action planning tools empower teams to understand survey results, create targeted plans, and act on strategies that will increase engagement, performance, and retention.
- Action library: helpful list of suggested team actions to improve key focus areas
- My Engagement (ME) Report: personalized engagement report with actionable recommendations for every employee
- Lightweight 1-on-1s: tools to drive manager-employee engagement discussions
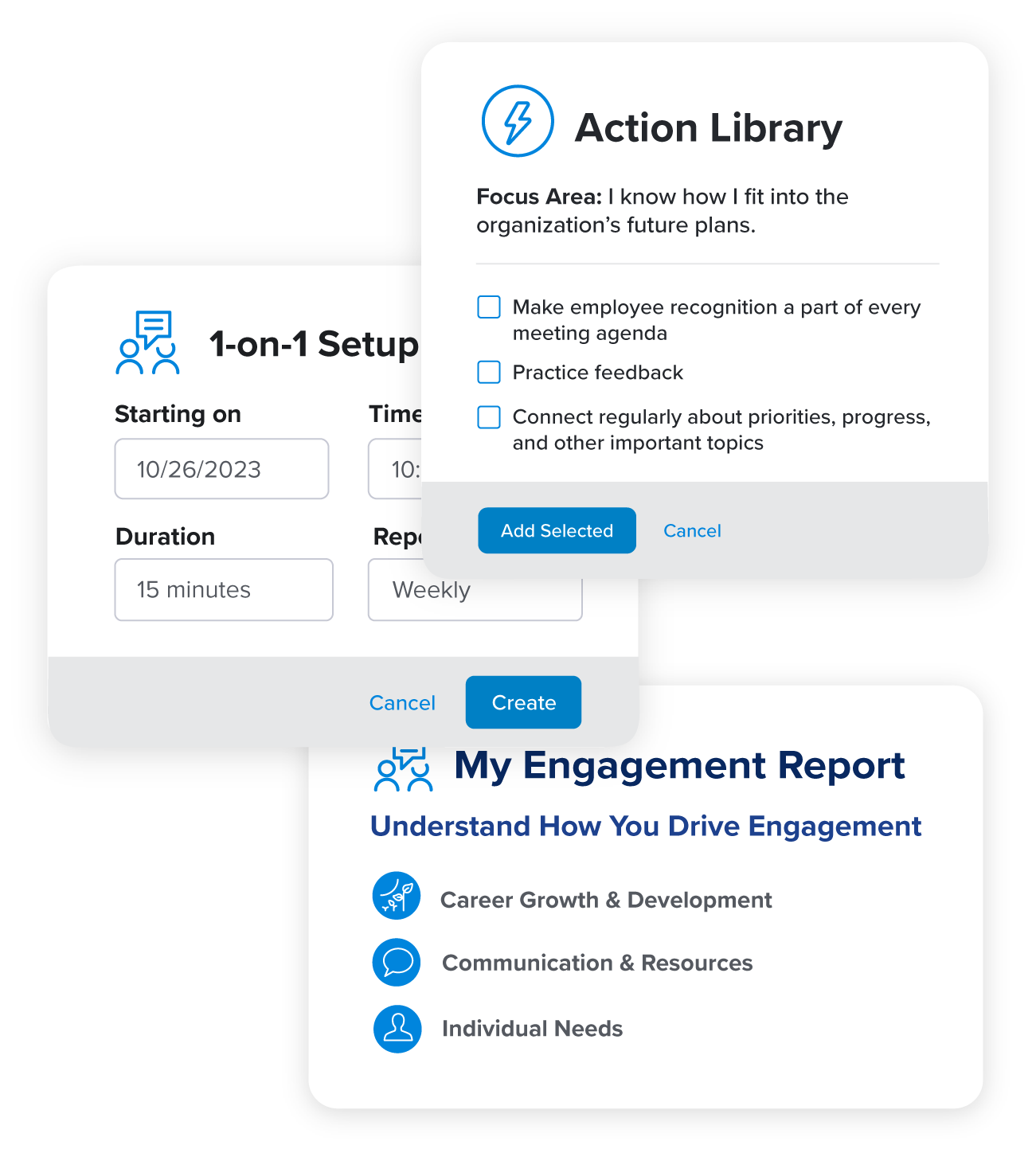
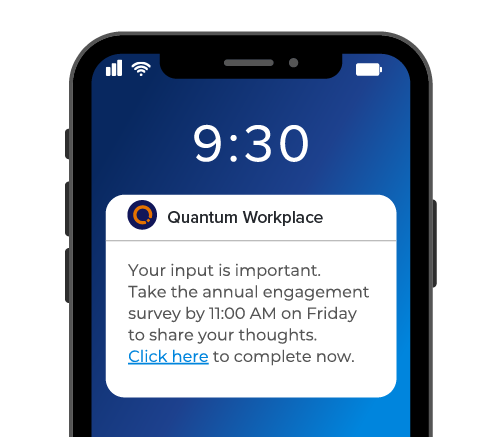
Reach every employee – wherever they are
Don’t choose employee engagement survey software that makes capturing feedback from a dispersed or deskless workforce difficult. You need solutions that can reach, activate, and empower employees who are always on-the-go with regular surveys.
- Accessible options: desktop, kiosk, and SMS texting functionality
- Automated invites & reminders: technology handles the administrative work, so you don’t have to
Uncover the meaning behind employee comments
Still reading through employee survey comments one-by-one? Quantum Workplace’s AI-powered Narrative Insights is an award winning solution that summarizes your comments in seconds – revealing underlying themes and sentiments, surfacing sensitive topics, and highlighting where you need to dig deeper.
- ✨AI-powered Smart Summary: secure, award-winning feature that summarizes thousands of comments instantly
- Theme & sentiment analysis: automated comment tagging that shows comment themes and sentiment at a glance
- Keyword detection: alerts for sensitive keywords, so you can follow-up proactively
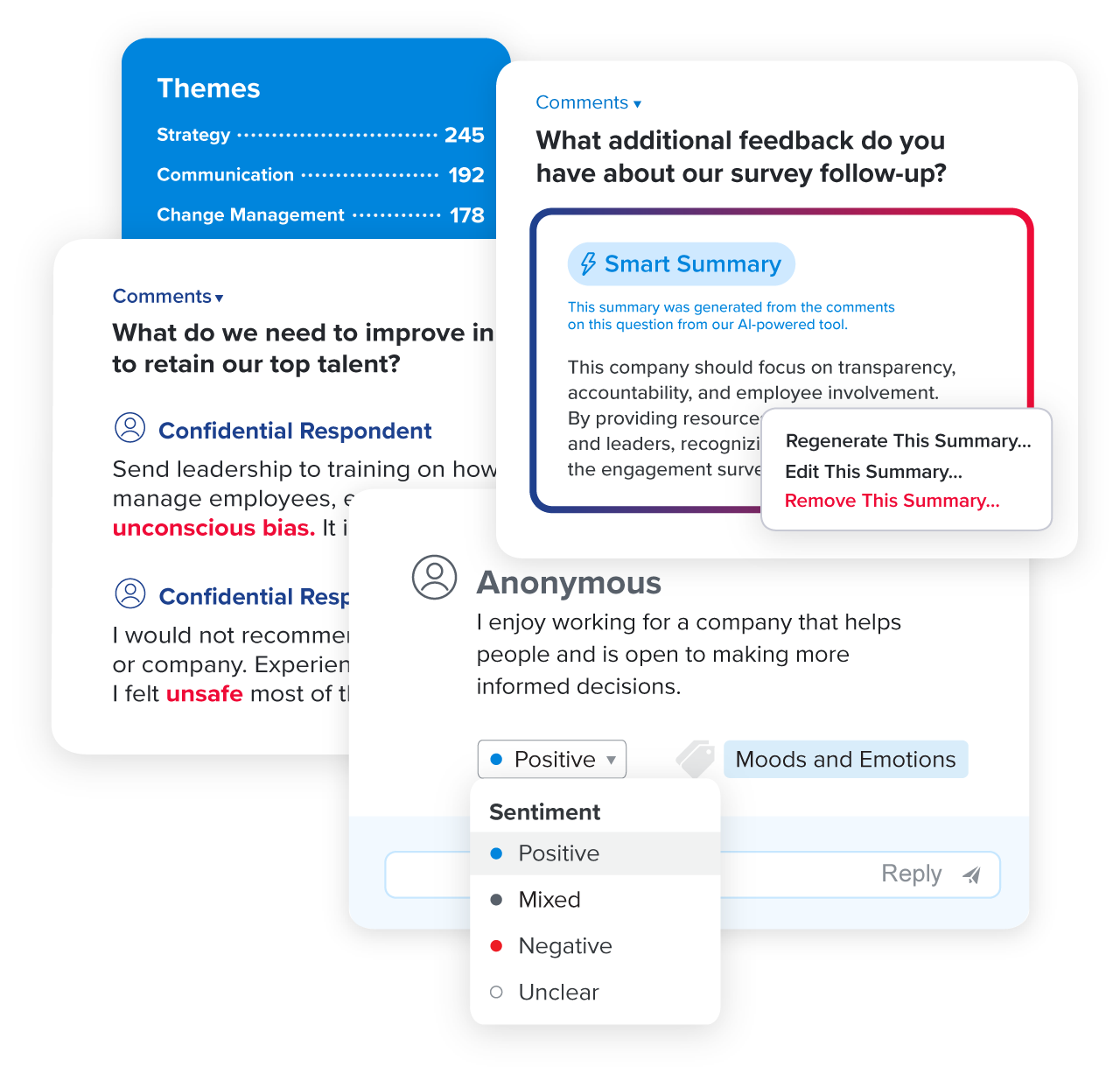
Find out why customers love our employee engagement survey tools.
“Quantum Workplace's employee engagement survey software helps us to seek employee feedback, shape our engagement programming, [...] and better achieve our employee needs and goals for engagement and culture. Our Frontliners are the reason we do everything we do. At Frontline, we leverage our survey to gain insight to enable our employees and our business to flourish.”
Jodi Dickinson
Chief Human Resources Officer at Frontline Education
Employee Engagement Survey Providers FAQs
What is an employee engagement survey?
Employee engagement surveys help organizations measure employee engagement and improve workplace culture to make it more tangible. They help organizational leaders:
- Uncover the meaning behind their employees’ experience
- Make employee insight actionable
- Implement changes that move the needle
A traditional employee engagement survey happens once a year and includes all employee voices. This type of employee survey is helpful in understanding employee engagement trends across the organization. It helps create organizational, team, and demographic benchmarks to track nuances over time.
Why should we conduct an employee engagement survey?
Employee engagement surveys (and the insights and strategies that come from them) can have a huge impact on business success. With employee sentiment, trending data, market benchmarks, and robust reporting, an engagement survey helps you:
- Understand where your company excels
- Shed light on where you need to improve
- Give every employee a voice
- Help connect the dots between employee engagement and your bottom line
- Build employee trust
- Compare and contrast among different team members and groups
- Drive meaningful action and smarter people decisions
- Capture actionable feedback that helps you navigate change and boost engagement
- Help you cultivate a competitive and engaging company culture that retains top performers
How do I design an employee engagement survey?
Developing an actionable employee engagement survey involves thoughtful survey design. Our employee engagement experts suggest staying focused on scientifically validated employee engagement outcomes and drivers and including trackable employee demographics.
Here are 21 expert-recommended employee engagement survey questions to include in your employee survey, including questions about:
- Employee engagement outcomes
- Career growth and development
- Communication and resources
- Individual needs
- Manager effectiveness
- Team dynamics
- Trust in leadership
- Future outlook
- Diversity and inclusion
What should I look for in employee engagement survey analytics?
To truly understand the meaning behind your results, you need to review your employee survey analytics in a thoughtful and systematic way. There are a few ways you can start to slice and dice your survey data for deeper insights:
- Evaluate and prioritize by favorability
- Use heat mapping to compare favorability by demographic
- Identify and evaluate keywords and narratives within your survey comments
- Use industry survey benchmarks
- Empower your managers to analyze their team results
- Help your employees understand their role in engagement
How do I take action on my employee engagement survey results?
Think of your employee engagement action plan as your strategic roadmap for employee engagement. It should outline specific initiatives and activities that your teams believe will help improve engagement—based on insights from your engagement survey results.
An employee engagement action plan typically includes:
- Key areas of focus based on engagement survey results
- Specific goals and objectives meant to drive progress
- Action steps required to achieve those goals
- Who is responsible for implementing action steps and by when
- Expected outcomes and metrics for measuring progress
How do I choose the right employee survey platform?
With so much to consider when evaluating which employee engagement survey platforms are right for your organization, it can be helpful to have a guiding light to make a clear decision.
Our guide to choosing the right employee engagement survey tool walks you through 12 questions to ask when evaluating survey software:
- Can you describe your engagement methodology?
- What does the survey launch process look like?
- How will you help me drive participation and response rates on my survey?
- What reporting capabilities exist? Are there benchmarks available?
- What options do I have when it comes to support?
- What other key features, resources, and content will I have access to?
- Does this employee engagement software integrate with my HRIS?
- How do you help my managers understand engagement and take action?
- Does your software integrate with other engagement tools?
- What options do I have in terms of customization?
- How do you keep your employee engagement solution up-to-date with the latest engagement trends?
- What other types of surveys do you offer?
What makes Quantum Workplace’s employee engagement survey software different?
Measuring and improving employee engagement easily and accurately requires a partner you can rely on. For the last 20+ years, our customers have trusted us to deliver reliable expertise, technology, service and results as one of the top employee engagement survey providers.
We help you uncover meaning.
We're more than a survey tool. Our scientific approach to survey design, research-backed templates, personalized engagement analytics, unparalleled benchmarks, and smart AI help you understand the meaning behind your employee voice data.
We make action easy for everyone.
The real power of your employee surveys is not in the surveys themselves, but in the action you take as a result. We make action easy for everyone — so leaders, HR, managers, and employees can all play their part in driving employee engagement.
We’re a partner you can rely on.
You’re on a journey – and we’re with you every step of the way. You can rely on our dedicated team to provide strategic guidance, ongoing coaching, timely customer support, and safe and secure practices your programs need to be successful.
EMPLOYEE SURVEY SOFTWARE
Every tool you need to quickly move from engagement insight to action.
Pulse Surveys
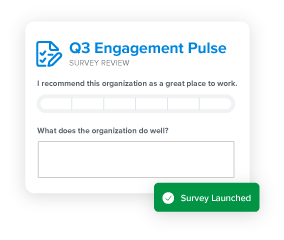
Get a pulse on what employees are feeling at any given time. Collect employee feedback efficiently on any topic.
Lifecycle Surveys
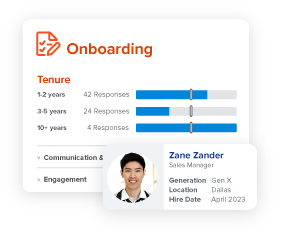
Assess and improve employees’ experience at every phase of their journey. Uncover insights at critical moments and milestones.
Analytics
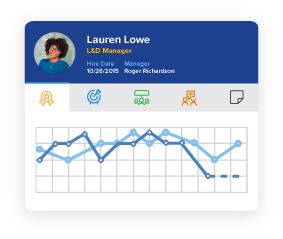
Understand the state of engagement and empower action with on-demand insights. Make sense of complex people data at-a-glance.
Benchmarks
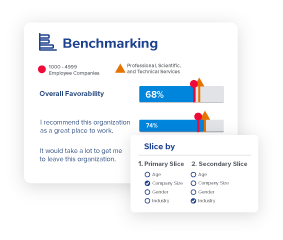
Get context that builds confidence with best-in-class comparisons. Compare your engagement results to similar organizations.
Action Planning
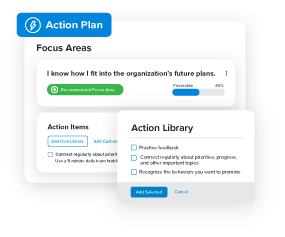
Create visibility and accountability for post-survey action. Reduce time-to-action, and increase impact. Enable managers to own and follow through on action items.
1-on-1s
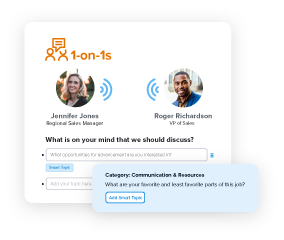
Strengthen manager and employee relationships through meaningful, ongoing conversations. Facilitate lightweight manager-employee 1-on-1s.
Want to see our employee survey tools in action?
Schedule a demo with one of our employee engagement experts today!
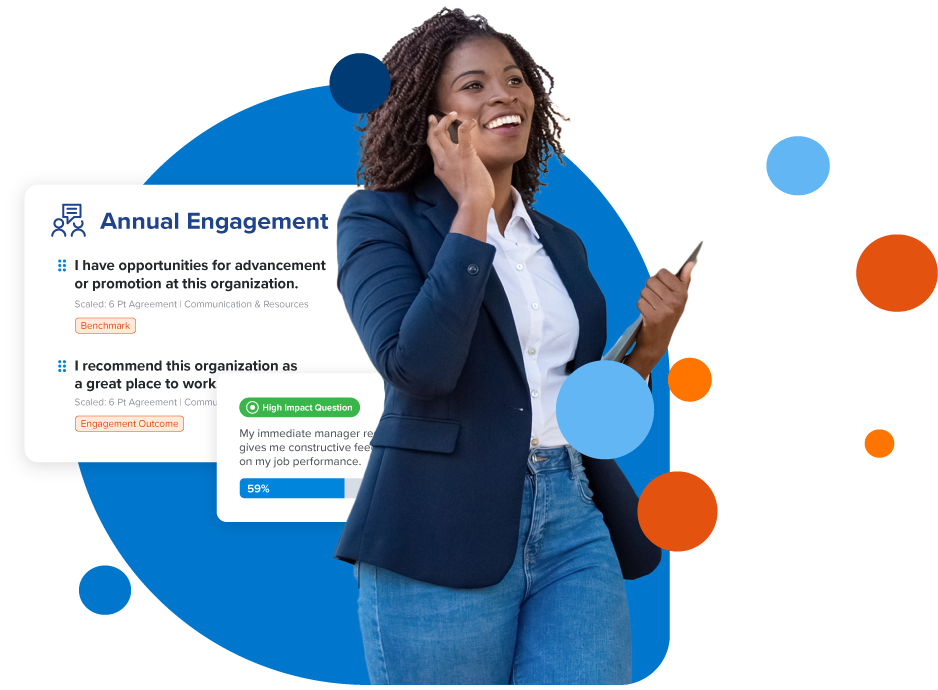